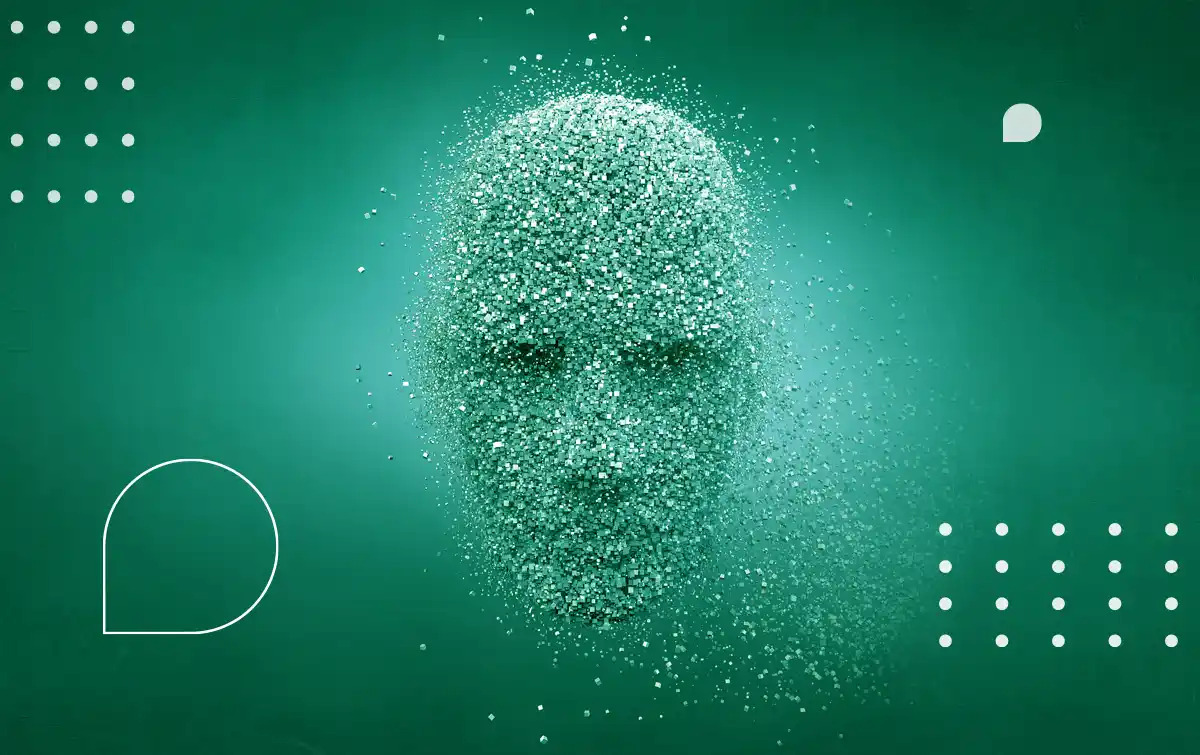
A New Era of Efficiency: How AI Can Boost Your Enterprise Architecture
Artificial Intelligence, and more specifically, Deep Learning technology, has the potential to disrupt the way businesses operate. Enterprise Architecture, an approach for managing the continuous transformation of companies, is starting to integrate this technology. How? Here are some insights.
In today’s fast-paced and ever-changing business landscape, enterprise architecture (EA) has become a crucial tool for organizations to stay competitive and efficient. By designing and optimizing the structure and processes of an enterprise, EA can help businesses align their goals, resources, and technology to drive innovation and growth.
However, the complexity and scale of modern enterprises often pose significant challenges to EA practitioners. Traditional EA planning and implementation methods may need help to keep up with the speed and diversity of business operations and IT systems. That’s where artificial intelligence (AI) comes in.
As a powerful technology that can automate, analyze, and optimize complex tasks and data, AI can potentially revolutionize the field of EA. By leveraging AI capabilities such as machine learning, natural language processing, and predictive analytics, EA professionals can gain new insights into their organization’s performance, risks, and opportunities. They can also develop more agile and adaptive EA strategies that respond to business environment changes and customer needs.
Innovation: How is AI used in Enterprise Architecture?
Enterprise Architecture is a method of continuously transforming organizations that must constantly adapt to their ecosystem: regulations, customer expectations, new technologies, etc. This collaborative method is based on a knowledge graph that describes the company’s constituents and their interactions and on a software governance tool to frame and orchestrate the transformation while remaining agile.
In the field of EA, classical algorithms are used to perform impact analyses, scenario comparisons, or the analysis of the propagation of incidents within the company’s structure. But as one might imagine, a business is a complex, non-linear system involving many variables. And for such a system, it is difficult to model a priori the equations of its behavior and the rules which govern its evolution.
Artificial intelligence, especially Deep Learning, plays on different levels of abstraction – hence the notion of depth – to extract meaning from data and produce a result while freeing itself from knowing the equations that govern the system.
Here are some fields of application of AI and Deep Learning relevant to EA:
Image recognition
This allows you to photograph drawings to convert them into structured models instantly. Models can be a process, data, application structures, models, computer networks, etc. The conversion of the drawing into a structured model makes it then possible to analyze it, for example, to know that such sensitive data is used within the framework of a given business process.
Linguistic translation
Many companies need to communicate internally in different languages: an official working language supplemented by local languages allowing each linguistic community to contribute to its native language. Instant or batch machine translation works very well to build a multilingual repository and facilitate internal communication and alignment.
Transformation of models
The particularity of any model is that it is not universal. Models are representations of reality designed for a particular use. We must transform the models to produce different views for various services. One may rely on a dual mechanism of formal graph transformation plus artificial intelligence to offer opinions that meet the concerns of multiple users in the company.
Natural Language Processing (NLP)
The (almost) natural language processing techniques are based on the semantic analysis of the question and its approximation to information from the frame of reference [via vector distances, for example – cf. Word2vec]. The provision of results via queries and reports makes it easier for all company employees to consult the data so that they understand its operation and structure and contribute to its transformation.
Data normalization
On the same principle as NLP techniques, data normalization makes reconciling terms and presenting data summaries possible. Typically, scan your IS in search of applications or technologies deployed across the company. The raw data will contain duplicates and variants – name differences, typos, minor versions of software, etc. – or insignificant components that will drown out the valuable information. It is, therefore, necessary to extract a clean, consolidated view, put it into perspective, and classify the management levers of the company.
Metadata and recognition of business patterns
New cloud tools embed more and more metadata, both in the context of big data and computer processing – descriptions of APIs, ETLs, etc. The semantic analysis of these metadata and their reconciliation with the information in the repository – portfolios of the company’s processes, functions, and products – facilitate the connection between the teams in charge of operational management and those in charge of new developments and continuous transformation. It is a crucial contributor to the success of agility on a scale.
Risk analysis of transformation projects
By collecting operational data from projects coupled with architectural data – scale, domain, complexity, ramifications, technologies, the scale of the transformation, timing, and resources … – we can try to predict the risk level of a transformation project. This risk is, of course, different from one company to another and considers many factors, such as that of its project culture, for example. Here, enough data from a company is needed to obtain a relevant signature that characterizes it.
Transforming Enterprise Architecture with AI-Driven Insights: A New Approach to Business Optimization
AI can be applied in various ways to enhance enterprise architecture projects, but the starting phase is particularly crucial. Using AI in starting an enterprise architecture program can have a significant impact compared to traditional methods. Without AI, identifying areas that need improvement in an organization can be time-consuming, resource-intensive, and prone to human error. For instance, manual data analysis may only effectively identify some bottlenecks, leading to developing an enterprise architecture program that must fully address the organization’s needs.
On the other hand, organizations can save time and resources by utilizing AI in the initial stages of developing an enterprise architecture program while gaining more accurate insights.
AI tools can quickly and efficiently analyze large volumes of data to pinpoint areas that require improvement, such as process bottlenecks or areas lacking automation.
By having a more precise understanding of these areas, organizations can develop a more effective enterprise architecture program that is tailored to their specific needs. AI can provide a competitive advantage by enabling organizations to build more efficient and effective enterprise architecture programs.
How Can AI Empower EA Architects in Their Daily Jobs?
AI can significantly assist EA architects in their daily job by providing valuable insights, automating repetitive tasks, and analyzing complex data in real time.
AI can help by delivering EA architects with best practices based on data-driven analysis. By leveraging advanced data analytics techniques, AI can identify patterns and trends that are invisible to the human eye, allowing EA architects to develop more informed and effective strategies.
Furthermore, AI can enable real-time, multi-source data analysis to assist EA architects in making prompt and informed decisions. For instance, in the automotive industry, AI can use advanced NLP to analyze unstructured data, such as vehicle specifications, internal communications, and maintenance logs, to identify key insights and trends.
With this information, EA architects can make informed decisions based on the current state of the business, supply chain trends, and production efficiency. By leveraging AI to analyze internal data sources, automotive companies can develop effective enterprise architecture strategies tailored to their specific needs and optimize their operations for success.
In addition to providing valuable insights, AI can automate repetitive tasks, freeing time for EA architects to focus on more strategic activities. For example, AI-powered chatbots can handle routine inquiries from stakeholders, freeing up EA architects’ time to work on more complex tasks such as designing and implementing new systems and architectures.
AI can also help EA architects generate essential documentation and report more efficiently using advanced natural language processing tools like GPT-3. This NLP tool generates high-quality text that closely resembles human writing, enabling EA architects to automate the report and document creation process.
This saves valuable time and effort, allowing them to focus on more strategic activities and provide excellent value to the business. By integrating AI tools such as GPT-3 into their workflow, EA architects can optimize their productivity, streamline their tasks, and become more effective.
Preventing Enterprise Architecture Failure: How AI Can Help Manage Complexity and Optimize Decision Making
Enterprise architecture projects can fail for various reasons, such as a lack of support from leadership, poor communication between stakeholders, and an inadequate understanding of the business’s needs.
However, one of the most common reasons for EA project failure is the inability to manage complexity effectively. With the rise of big data and increasing business complexity, EA architects face more challenges than ever.
This is where AI can play a significant role in preventing EA project failure. AI-powered tools can help EA architects manage complexity by providing insights and identifying patterns that would be difficult to detect using traditional methods.
For example, AI can help EA architects analyze large volumes of data from different sources and provide valuable insights into business processes and systems.
Moreover, AI can be a valuable asset to EA architects in identifying and anticipating potential issues that may impede project success. For instance, AI can flag potential risks and dependencies impacting project timelines, budgets, or scope. With this knowledge, EA architects can more effectively manage risk and prevent project failure.
Beyond risk management, AI can also enhance the decision-making process for EA architects. By providing timely and accurate insights, AI can help EA architects make informed decisions more efficiently.
With AI-powered tools, EA architects can evaluate various scenarios and predict their impact on the organization. EA architects can reduce the risk of poor decision-making by making decisions based on data-driven analysis rather than intuition alone.
Leveraging AI Techniques for Enhanced Enterprise Architecture Tools
Enterprise architects can leverage the power of AI techniques to enhance their tools and gain valuable insights into their organization’s architecture.
One such technique is neural graph networks (GNN), which can help EA architects analyze complex data and identify patterns and relationships between systems and processes. By leveraging GNN, EA architects can better understand the enterprise’s architecture and optimize its performance.
Machine learning (ML) recommendation systems can be a powerful tool for EA architects to make informed decisions confidently. By analyzing large volumes of data, ML recommendation systems can classify and recommend the best course of action based on current business needs and performance metrics.
The system can provide a confidence rate with each recommendation, enabling EA architects to evaluate the risk associated with each decision and make informed choices.
Strategy to Envision the Future of AI at the Service of Enterprise Architecture
It should be promising! As we can see from the examples mentioned above, Enterprise Architecture is not immune to the attractiveness of AI’s potential. We will see developments in its usage in the years to come. We are working on its application to the most difficult challenges for companies: continuous transformation and the design of the future-proof enterprise. But this requires a powerful representation (digital twin) of the company and large volumes of data, two drivers for new types of solutions.
We are also working on solutions to identify transformation opportunities and recommend transformation scenarios. For example, the identification and recommendations of migration strategies to the Cloud … To be continued!
Final thoughts on AI’s role in enterprise architecture
In conclusion, integrating AI in enterprise architecture can transform this critical field by providing valuable insights, enhancing decision-making, and improving the enterprise’s overall performance. However, addressing AI ethics, security, transparency, and explainability concerns is essential to ensure that AI is used responsibly and effectively.
By leveraging AI techniques such as graph deep learning, advanced NLP and language understanding, ML recommendation systems, image recognition, and pattern recognition, EA architects can optimize the enterprise’s architecture, reduce risk, and increase the chances of project success.
Ultimately, integrating AI in enterprise architecture is not a replacement for human expertise but rather an enhancement, providing EA architects with powerful tools to deliver value to the business more efficiently and effectively.